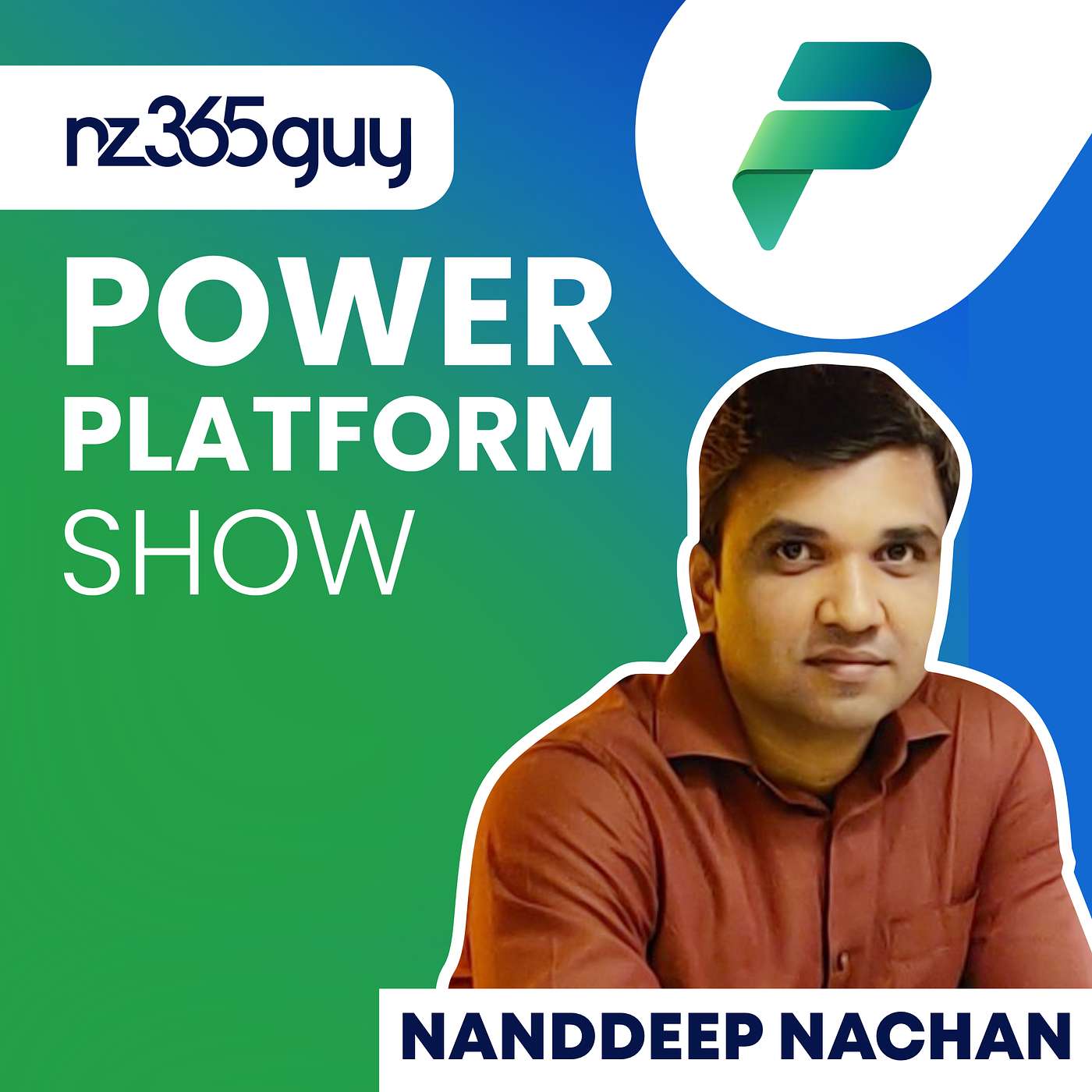
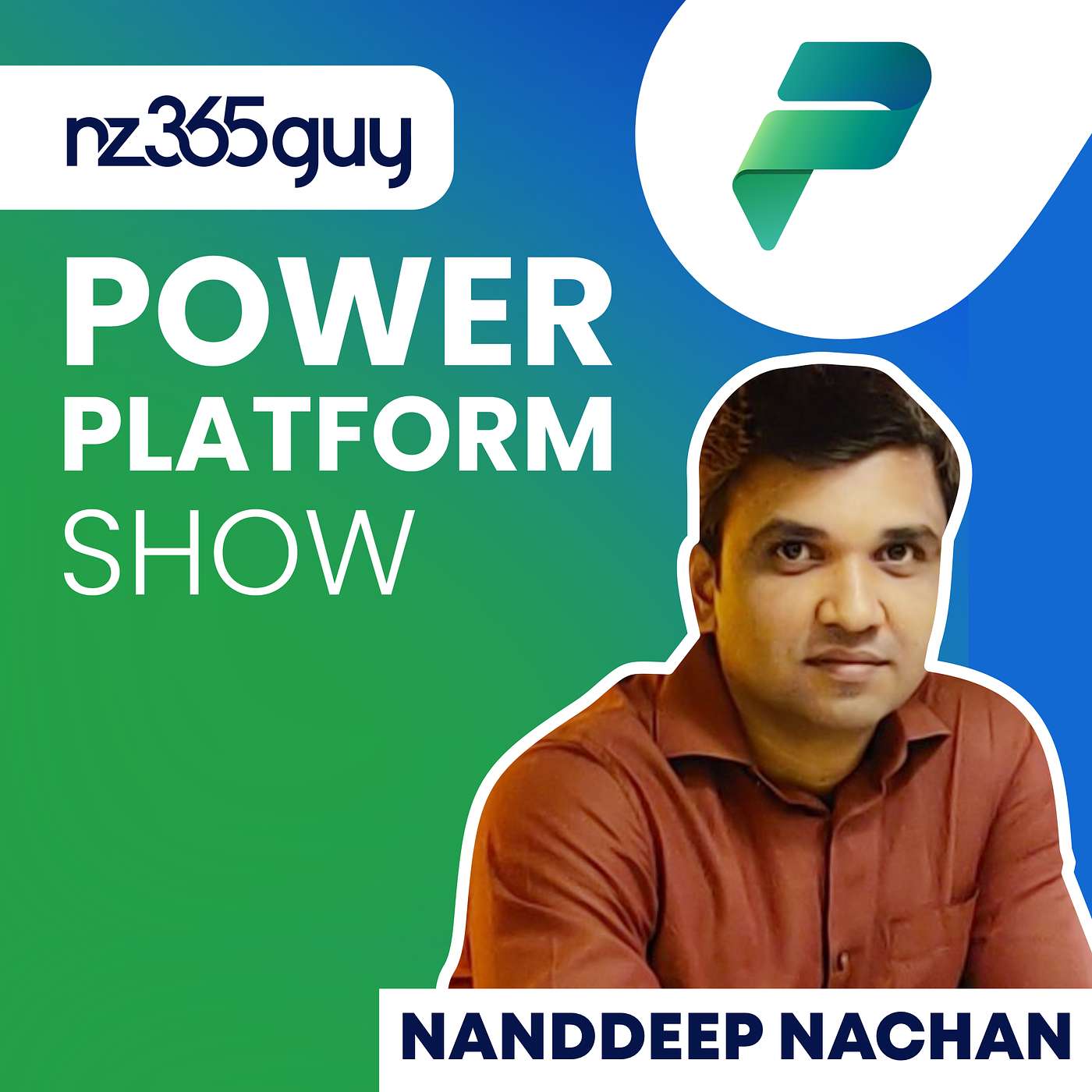
Building AI Solutions with Azure AI Foundry
Nanddeep Nachan
Get featured on the show by leaving us a Voice Mail: https://bit.ly/MIPVM
FULL SHOW NOTES
https://www.microsoftinnovationpodcast.com/680
Microsoft's AI landscape has evolved into three distinct categories: Copilot for Microsoft 365 (M365) applications, Copilot Studio for low-code chatbot development, and Azure AI Foundry (formerly AI Studio) for pro-code flexibility with AI models. Join Nanddeep Nachan on today's Power Platform Show to learn more.
TAKEAWAYs
• Declarative agents provide the simplest approach to extending Copilot functionality without complex licensing
• Teams toolkit in Visual Studio Code offers an easy way to create declarative agents using simple JSON configurations
• Copilot Studio gives business users a drag-and-drop interface for creating virtual assistants quickly
• Azure AI Foundry provides comprehensive tools for developers and data scientists building advanced AI solutions
• Retrieval Augmented Generation (RAG) pattern bridges the gap between LLMs and organization-specific data
• Contract management use cases demonstrate how AI can extract insights from millions of documents
• Graph RAG pattern enables "global queries" that deliver insights across entire document collections
• AI Foundry solutions can be deployed directly to websites, Teams apps, or Microsoft 365 Copilot
• Despite impressive personal productivity gains, many organizations still struggle to find compelling enterprise-level use cases for Copilot
🔴 Books:
➟ Mastering SharePoint Framework (BPB Publications), Amazon bestseller - https://www.amazon.ca/Mastering-Sharepoint-Framework-Easy-Follow/dp/938932887X
➟ Understanding Hybrid Environments in SharePoint 2019 (Apress) - https://link.springer.com/book/10.1007/978-1-4842-6050-0
➟ Up and Running on Microsoft Viva Connections (Apress) - https://link.springer.com/book/10.1007/978-1-4842-8606-7
This year we're adding a new show to our line up - The AI Advantage. We'll discuss the skills you need to thrive in an AI-enabled world.
DynamicsMinds is a world-class event in Slovenia that brings together Microsoft product managers, industry leaders, and dedicated users to explore the latest in Microsoft Dynamics 365, the Power Platform, and Copilot.
Early bird tickets are on sale now and listeners of the Microsoft Innovation Podcast get 10% off with the code MIPVIP144bff
https://www.dynamicsminds.com/register/?voucher=MIPVIP144bff
Accelerate your Microsoft career with the 90 Day Mentoring Challenge
We’ve helped 1,300+ people across 70+ countries establish successful careers in the Microsoft Power Platform and Dynamics 365 ecosystem.
Benefit from expert guidance, a supportive community, and a clear career roadmap. A lot can change in 90 days, get started today!
If you want to get in touch with me, you can message me here on Linkedin.
Thanks for listening 🚀 - Mark Smith
00:27 - Welcome to the Power Platform Show
00:59 - Introduction to Azure AI Foundry
02:00 - Nandip's Journey to Independence
04:41 - Microsoft's AI Ecosystem Explained
06:28 - Declarative Agents: The Simple Solution
10:31 - Copilot Studio vs Azure AI Foundry
17:08 - RAG Pattern and Contract Management
23:28 - Global Queries and Knowledge Graphs
25:49 - Surprising Limitations of Enterprise AI
Mark Smith: Welcome to the Power Platform Show. Thanks for joining me today. I hope today's guest inspires and educates you on the possibilities of the Microsoft Power Platform. Now let's get on with the show. In this episode, we'll be focusing on Azure AI Foundry, formerly known as AI Studio, Azure AI Studio, so we'll dive deep into the subject today. Today's guest is from Pune, India. He's self-employed and works as an Office 365 consultant and M365 consultant. He likes reading historical books and a big fan of Sherlock Holmes. He has authored his own books. We'll unpack that in a moment. You can find links to his bio and social media in the show notes for this episode. Welcome to the show, Nanddeep. Thank you.
Nanddeep Nachan: Thank you very much for inviting me. I guess it's my second time, but I do enjoy talking to you. Thank you very much.
Mark Smith: Yes, yes, as an MVP, you've been on the MVP show that we've discussed in the past, but I'm interested to, of course, to drill into this topic of the AI foundry the Azure AI foundry with you. Before we do, let's get a bit of background. What have you been up to most recently? What's happening in your world?
Nanddeep Nachan: What have you been doing in this past year Right At least I started doing my own business this year, so at least I've got almost 20 years of experience. I've been mostly working into various organizations, but this year I started my own consulting thing, which is a new adventure for me, but again, I found it very interesting because if you are a consultant, obviously you get a chance to work with more people, you explore your more network as well as to expand your boundaries. When you have a limited kind of an exposure, when you are working in the into the organization, probably you just have a trail around you and then you just talk to only a few certain people who you know, whom you work with. But when you come out of it, when you start your own business, obviously you expand the boundaries. So that is what I'm doing right now I'm exploring this world. I'm just into this consulting just two months old, so quite new here but yeah, I'm expanding my boundaries, I'm talking to various people and finding the opportunities from there as well, so, which is a shift that I'm observing during the end of this year. But yeah, it is a challenging as well as an interesting one.
Nanddeep Nachan: What made you go independent? Yeah, I mean at least, as I said, I worked almost for 20 years into the IT industry. So I just never thought like, for example, if I don't do it right now, then when am I going to do it? So I just thought, okay, this is the correct time, let us go ahead explore various things, because in 20 years obviously you do get much exposure, much knowledge like how the things work. You do get your own network as well. So I just thought probably this is a good time to go ahead, start own consultancy and take from there. But yeah, I guess I do have a good kind of support from the MVP community as well as from the community in general. So yeah, so far, this two months whatsoever, I'm into this new role, I'm enjoying it.
Mark Smith: Excellent, excellent. And you talk about your network. There is that where your business is coming from at the moment. It's the people that you've built relationships with over time. Yes, yes, mostly like.
Nanddeep Nachan: I have been into this community for, I would say, more than 7-8 years active into the community. Otherwise earlier I was just a passive listener just listening to these stuff and, as well, just reading out. But since the past six to seven years I am mostly active into this. So I have grown into the network and again mostly from there as well. I'm getting some good opportunities to work, maybe collaborate with a few of the colleagues, as well as trying a few things on my own.
Mark Smith: Nice, nice. So today I want to talk about Azure AI Foundry and of course we're what? Two weeks on now from Microsoft Ignite recording this in December, microsoft renamed their product, which was AI Studio, to Azure AI Foundry. Microsoft renamed their product, which was AI Studio, to Azure AI Foundry.
Mark Smith: And when I look at this AI landscape from Microsoft, I see it in three distinct buckets. I see Copilot M365, which is designed to work with all the Microsoft 365 suite of tools that Microsoft provide under M3 and M5 licensing. If you add the Copilot SKU on, you get that all lit up PowerPoint, excel, sharepoint, of course, and various others. Then in the second stream I see is Copilot Studio from Microsoft right, which back in the day was Microsoft Power Virtual Agents and that's now been extended and ultimately you can build agents on that platform and I think there's going to be a big play for that. I think over 1,400 connectors to other ecosystems where you can build applications in a low code type of experience you know that we always had in the Power Platform arena. And then we move into this Azure AI Foundry which for me, the way I look at it is, it's the pro code area. It's where you kind of have unlimited flexibility in how you can take models and apply them to your work. How do you explain it?
Nanddeep Nachan: Oh right, as you said correctly, right now we are not just limited to using SharePoint, but at least we are finding out various ways to expand the SharePoint or at least the co-pilot, or maybe extending the co-pilot. So, as you said correctly, we have got at least three permanent kind of options. First one is obviously declarative agent, which is a very new one. Again, we used to call it a declarative co-pilot, but again after Ignite we call it as a declarative agent. Again, there are changes as well. Then we do have the AI Foundry, azure AI Studio, which is Azure AI Foundry right now, and Cooperate Studio, for example. If we go into each one of those one by one, probably I will choose the very simple one At least. You might be amazed that I'm not choosing very simple one as the Cooperate Studio, but I will choose very simple one as the declarative agent. Okay, because when you are developing something on top of co-pilot or whenever you're trying to extend the co-pilot, obviously the first option that comes to our mind is co-pilot studio. But I will say that after ignite, when the declarative agent got introduced, it bit of changed the landscape. So from the easiness perspective, I will say declarative agents is my own favorite because those are very much simple to consider, simple to use, without taking into complexity of licensing configurations, all those things. It is very, very simple. So, in simple words, like declarative agents will let you create a customized kind of a copilot for Microsoft 365 by simply starting on what you want that to do. So these are the agents that use the same technology as a Microsoft copilot but they allow you to add up to the business need very easily and then again, by using the declarative copilot, you can improve the collaboration, boost the productivity and all those kind of things. It is a newer approach which just help us to configure and deploy those AI copilot across Microsoft 365 applications.
Nanddeep Nachan: What are the benefits of this declarative copilot or declarative agents? They are very much easy to use because they just run onto these declarative approach. Now that, for example, if we try to segregate that, we earlier had the imperative approach wherein we need to define the path that co-parate should take. Let's say, for example, if this is a response that we are getting from the AI model, then again, for example, this is a prompt that we get from the user. In that case, what should be our next step that we used to define, which we call it as the imperative kind of a model, but if decorative agent, they take the entirely different path, called as a decorative approach, which simplifies your development process and make it more accessible even to the non-developers, so that they can enable the users to define the agent behaviors. So, in simple terms, I have been using the approach of using Teams toolkit to create the agent behaviors. So, in simple terms, I have been using the approach of using Teams toolkit to create this declarative agent. It is pretty much simple that there is just one file. In that one file, you need to define your prompt and you just need to define what should be the behavior for that agent and that's it. It will do the rest of the tricks for you. And again, there is extensibility on top of it as well. For example, if you want to get connected to other sources like SharePoint or OneDrive, wherein most of the organizations are using them for storing the data, or even to Microsoft Teams, or even to any of the third-party systems using REST APIs, those are very much supportive. So that's the reason I said like after Ignite.
Nanddeep Nachan: Personally, my favorite choice to go ahead for creating the copilot or extend the copilot has become a declarative copilot. Yeah, before Ignite. If you could have asked me this question, I could have directly went for Copilot Studio, because it was a very simplistic kind of an approach. But this is kind of a shift that I see after Ignite, because at least before Ignite this concept was there, using declarative co-pilots which they just have renamed to declarative agents. But since that capability was not available into everyone's tenant at least in my tenant I did not able to see the power of it. But now that's when I'm experiencing that power, at least whenever there's an option to get two simplified things the declarative agent, it is my first choice.
Nanddeep Nachan: Okay, so let's talk about the second part, which is the copilot studio. Okay, so again, copilot studio is pretty much simple thing wherein you can use the low codecode, no-code kind of a functionality or flexibility. So even having much knowledge about the technology, you can just simply focus on the business logic and from there you can get started creating the co-planet. So again, it gives you the drag-and-drop kind of an approach, or maybe just the topic kind of a thing, wherein you will be able to define how should be the flow of your component and then you will be able to perform all those things. When we go for the third approach, which is ai studio or ai foundry, which is recently renamed, when we get the entire flexibility okay, so at least it is probably I will not say it is for everyone At least you need to have some basic understanding of the AI concept regarding how the models work, how should we use the prompt engineering and all those kind of things, because if you directly jump into the AI studio or the AI foundry and start explaining the things, you might see it very overwhelming.
Nanddeep Nachan: So in that case you need to have some basic information or some basic knowledge about how to use those AI models.
Nanddeep Nachan: And again I will say, at least you need to be very much on the top of using your models, of where it should be deployed, how it should be deployed and many more technical things.
Nanddeep Nachan: So from that reason, at least for the business people, I will say still the good choice to go ahead with creating the co-pilot is Microsoft Co-Pilot Studio and, for example, if you have basic understanding, whether you know how the LLM works, what is prompt engineering, how the parameters work with those, then obviously you can go ahead with the AI Foundry.
Nanddeep Nachan: So one thing that I will say is choosing the right platform for your development of the AI is very much crucial because now that we are into the world like, where we increasingly embrace the AI technologies, so the tools like corporate studio or this Azure AI Foundry they have emerged very kind of a significant, kind of a clear scene in the market.
Nanddeep Nachan: There's both platform offer unique capabilities which are tailored to different user needs and scenario. Mostly, as I said, if you are looking out to create a solution very quickly so that you will be able to develop them for your customers, with some drag and drop interface which will allow you to build your chatbots or virtual assistant very quickly, go for Microsoft Go Palette Studio, for example. If you're looking out for interface which will allow you to build your chatbots or virtual assistant very quickly, go for Microsoft Go Palette Studio, for example. If you're looking out for a very comprehensive kind of a platform which is designed for developers or maybe for data scientists to build, train, deploy your AI models so that you can leverage the capabilities of Azure Cloud and then develop your advanced AI or machine learning kind of solutions, then obviously AI Foundry is your option. So, yeah, these are the three options, prominently declarative agent, copilot Studio or AI Foundry, but again I will say after Ignite. Declarative agent is one of my favorites to go ahead with.
Mark Smith: So let me get this right. Declarative agents are accessible or buildable from within Copilot directly. Is that right? Is that the UI that you use, correct?
Nanddeep Nachan: So there is a UI as well, but at least I have been using Teams toolkit for creating those. So if you have got Teams toolkit, which is an extension inside of Visual Studio Code, and when you create a project out of it, they just give you the option to create a copilot or agent Right now it is renamed to agent. Then, once you select the agent, there are again two options. Like you can create it from any of the API or you can create that from scratch. From scratch is again the same kind of a thing where you will be able to define your prompt as well as you'll be able to define the location or the data source from where you want your copilot to get the data from. If, in case, you do not specify any location, then it is the general kind of an internet information which is available to the copilot. So let's say, for example, you're just building a corporate agent which can test the knowledge of geography for any of these given users. In that case you can just mention into the system message of that model, just saying that okay, I want to create this kind of an agent which can help test the knowledge of geography or history, and then, once you start conversation with that agent, it will start asking you those kind of questions and again.
Nanddeep Nachan: Secondly, it is a different kind of structure wherein you can start building your own components, and again, data source is one of the components there. So you can define like, okay, I don't want to probably get the information from the internet, but I want to restrict my scope to just a SharePoint site or maybe any of the Graph API or any of the REST API, and even from there it can surface the information. So, yeah, again, there you need a basic kind of configuration information. But if you just at least know that JSON, even that is fine you will be able to create those very easily. So even it is very much easier than Copilot Studio, just that you need to know the JSON, how to do that. And the advantage is that probably you don't need the license, as the Copilot Studio needs.
Mark Smith: Okay, interesting, interesting little work around there. What type of use cases are you seeing at the moment? Or what have you worked on where you know specific things that people are wanting to achieve using not just declarative agents but any of the co-pilot through to foundry use cases? What have you seen so far?
Nanddeep Nachan: Mostly I have seen people are building the use cases around RAC pattern, the travel augmented generation, for example. If I start from very basics, we do have those various models available with us a large language model, specifically GPT 3.5, 3.5, turbo, gpt-4, even we have went up to GPT-4 as well. They have their own training data up to which they know the information, what is happening in this world. But after that probably they are not being trained on At the same time when we want to use this kind of capability of this large language model into our organization. Obviously, since these are kind of an openly trained model, they do not have any insights into our organizational data. So there are two things now. Like we need to bridge that gap between the last trained data of that large language model and the second use case is like we need to bring our own data so that we'll be able to ask the questions to the large language model based on that data. So to fill that gap we have got something called a retrival augmented generation. That is lag pattern. So what we do simply is like we do not train that model as such, but at least we make those model capable or aware of the information that we have got inside of our organization. So, for example, that could be anything, it could be just the documents, or even it could be any of the information that we can vectorize, store them into an index and then again give it to those large language model. Then, by looking at that index, those large language models can help us to give the information.
Nanddeep Nachan: One of the use case which I recently had built. So again I will start with the simplistic one. There the first thing was about the contract management kind of a system. So, for example, there are various organizations who have a huge number of contracts with various vendors as well as maybe within their departments or even worldwide. So again there are various organizations who have got even billions of those records of their contracts as well. So even there are few contracts which have been running over the years, which are kind of handwritten, where we have information or maybe scan kind of contracts. So then the use case starts like we do have that data into, not digitally probably, but just the scan kind of information. Or maybe you can take a photo of that contract and then make it as a part of your AI system. So the story starts from there. We get those kind of contracts and from there we bring that into our ecosystem. So there we use the basic capabilities of AI, for example OCR or maybe image to text kind of capabilities, to get text out of it. Then again, once they are into the text that is where the story begins for RAG we provide that information or we vectorize that information provided to the large language model and from there we can talk to that information. So what can happen is you can ask who is the point of contact for Contoso contract and then it can surface that information by looking at that index and give you the information.
Nanddeep Nachan: But in the recent days, even though it does not end here, there has been a few things like when we talk to these kind of models there is a generic kind of information or I would say like there are two set of questions that we can have when we are specifically trying to build this kind of use cases. So first thing is local queries, wherein we are just trying to get the information which is built on that index. So let's say, for example, who is the contact person of Contoso contract? Or print of the Contoso contract end okay, because this is where the index has been built. So let's say, for example, we have one Contoso contract document and inside that document we have this information in place. So we'll be able to easily get that information by looking at that index. Second query comes when it is called as a global search or global query. So global query, that means we are holistically trying to get the information from the information which is available in all of the index data. So what is meant by that is like, let's say, as I said, we may have millions of contracts. I can just ask a question like how many contracts are ending in January 2025? Or maybe how many contracts are ending end of this year? We do not have this information indexed anywhere, but this is holistic information which is available on all of the index which is available. That means we cannot directly expect that information to be available in any of the documents which got indexed.
Nanddeep Nachan: And then we can ask that information. It has to be generated via something known as a knowledge graph. Those knowledge graphs are again built by using another pattern, which is developed by Microsoft as well, which is called as graph rack. We already had that rack and again we are introducing these graph patterns, that graph rack. What it does is it creates that knowledge tree around that or knowledge base around that. Then again, all those dots are connected to each other. What happens via that is like it knows, like, for example, when I have a look at the contract, that means these are the contracts which are related to each other, and then it creates that entire knowledge graph around the information which is there and then, using that in knowledge graph, we will be able to ask the questions about the holistic information of that. So why that? What can happen is like I can ask the high level kind of information as well, like how many contracts are ending in next 14 months, or maybe like how many active contracts that we have got into a system. So these are the places which I'm working, which is a very interesting thing.
Nanddeep Nachan: Again, if you are trying to build this kind of a system where you will be able to also ask the holistic kind of a question, then obviously AI Foundry is only your option. You unfortunately, will not be able to do that with declarative corporates or even with the corporate studio, because there you need to bring your own graph, rack pattern, you need to build your own information database and everything. So yeah, at least there are pros and cons of using any approach, whether it is Declarative, cooperate Studio or AI Studio, but again, based on what fits your need, it is the best one. So you need to choose what kind of use cases that you are trying to solve for your business case and then you need to pick out the right options. But again, we do have a lot of options right now. In case you want to create a component, there are various options. And then again, one good thing which Foundry makes it available right now, is that you have built your own RAC pattern.
Nanddeep Nachan: Obviously, from the Foundry Studio itself, you will be able to deploy that solution directly to a website or secondly, to Teams as an app, or even thirdly, to the co-pilot. So even from there, you will be able to deploy directly toly to the co-pilot. So even from there you will be able to deploy directly to the Microsoft 365 co-pilot. So that solution is available as a Microsoft 365 co-pilot as well. So right now, these two options the deployment to Teams as well as Microsoft 365 co-pilot they are in preview but they work very perfectly.
Nanddeep Nachan: So not sure when they will go GA. There might be some differences, but at least the functionality will continue to work. But yeah, that is the beauty of using AI Foundry and again, it is not working into its own shell. Microsoft have make it very generic so that, for example, if you want to develop something in Foundry, take it to the next level, probably into your own website or into Microsoft 365, it is still open. So it is not at all like when you are working in AI Studio or at AI Foundry, you are working in silos. It is not at all like that. You can anytime extend that to any of your platform where you want.
Mark Smith: Yeah, excellent, excellent. In the past year of working in this technology, what surprised you the most and I suppose what surprised you the most about using AI? And then, what limitations have you seen?
Nanddeep Nachan: Oh yeah, at least we have been using CoPilot specifically for the last two years. I guess so, oh sorry, I can CoPilot for for the last two years. I guess so, oh, sorry, I think, co-pilot for the last one or one and a half years or so. So mostly, like I have seen, we are using Microsoft Co-Pilot very effectively for translating the document, transcribing the things or even creating a meeting notes and everything. But even I see that there are many organizations who are still struggling to get to the right business case for Microsoft Co-Pilot, which is a bit surprising because Co-Pilot is very nice, it is very excellent because, for example, if I even compare myself when I was not using Co-Pilot versus when I'm using Co-Pilot, at least I feel OK, I'm very, very organized. So, at least on a personal level, it is giving you very flexibility to use the Copilot in very effective way. So, for example, let's say you are on a leave for five to six days and when you come back, so, for example, you can see a lot many conversations into your teams, as well as maybe hundreds or 200s of email, and then again, again, you need to go through that. Um, at least two, two days. We'll go into that that itself. But with copilot at least you can quickly summarize everything and maybe just within five to ten minutes you will be able to draft on your agenda, like what is the next thing that you should do? So thanks to copilot on that. So at least yeah, I mean least Co-Pilot is helping us to create that kind of an environment, at least for the personal level, even at the team level.
Nanddeep Nachan: But still it is a bit surprising for me, like even still, after using Co-Pilot for one or maybe even for two years, now at least we don't have any much enterprise-level use cases for corporate. But again, at the same time, microsoft have given good options for extensibility of that as well. But it is a place where I'm still thinking like we are a bit of missing some kind of use cases. So we do have various levels of corporate, like we do have corporate for sales, corporate for finance, corporate for developers. So we do have various levels of corporate, like we do have corporate for sales, corporate for finance, corporate for developers. We do have everything, but maybe, for example, holistically, if we think around, an entire use case for an organization. Probably we are a bit of missing there.
Mark Smith: Yeah, interesting, Nanddeep. It's been great talking to you. Thank you so much for coming on the show. Yeah, thank you very much. It. It's been great talking to you. Thank safe out there and shoot for the stars.
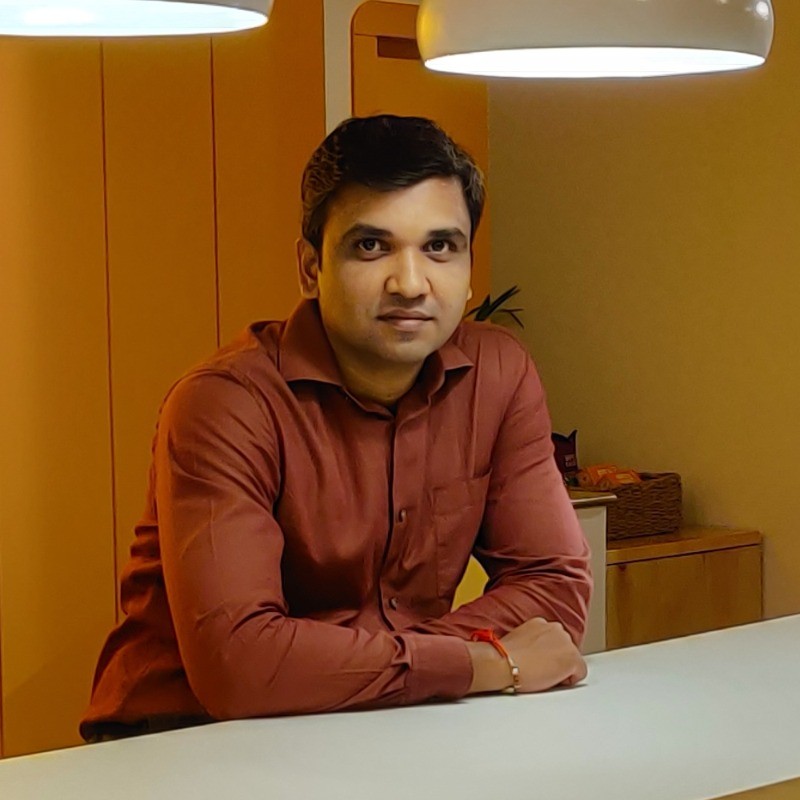
Nanddeep Nachan
Nanddeep Nachan is an independent consultant, 5 times Microsoft MVP (M365 and AI Platform), MCT, and TOGAF 10 Certified professional. He is a results oriented consultant with experience in Microsoft Technologies especially with Microsoft 365, Power Platform, and Microsoft Azure. Experienced in design, implementation, configuration, and maintenance of several large-scale projects.
Author of books:
➟ Mastering SharePoint Framework (BPB Publications), Amazon bestseller
➟ Understanding Hybrid Environments in SharePoint 2019 (Apress)
➟ Up and Running on Microsoft Viva Connections (Apress)